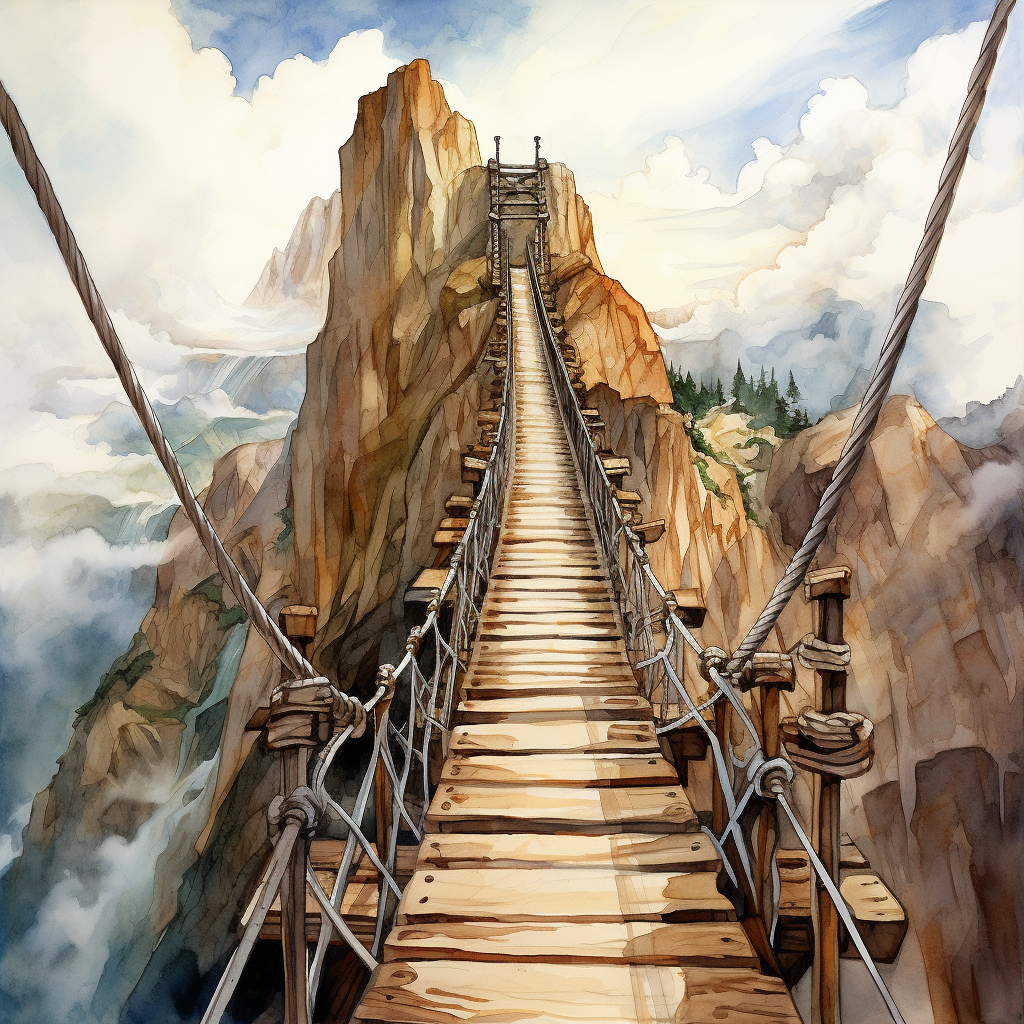
The Optimal Level of Optimization
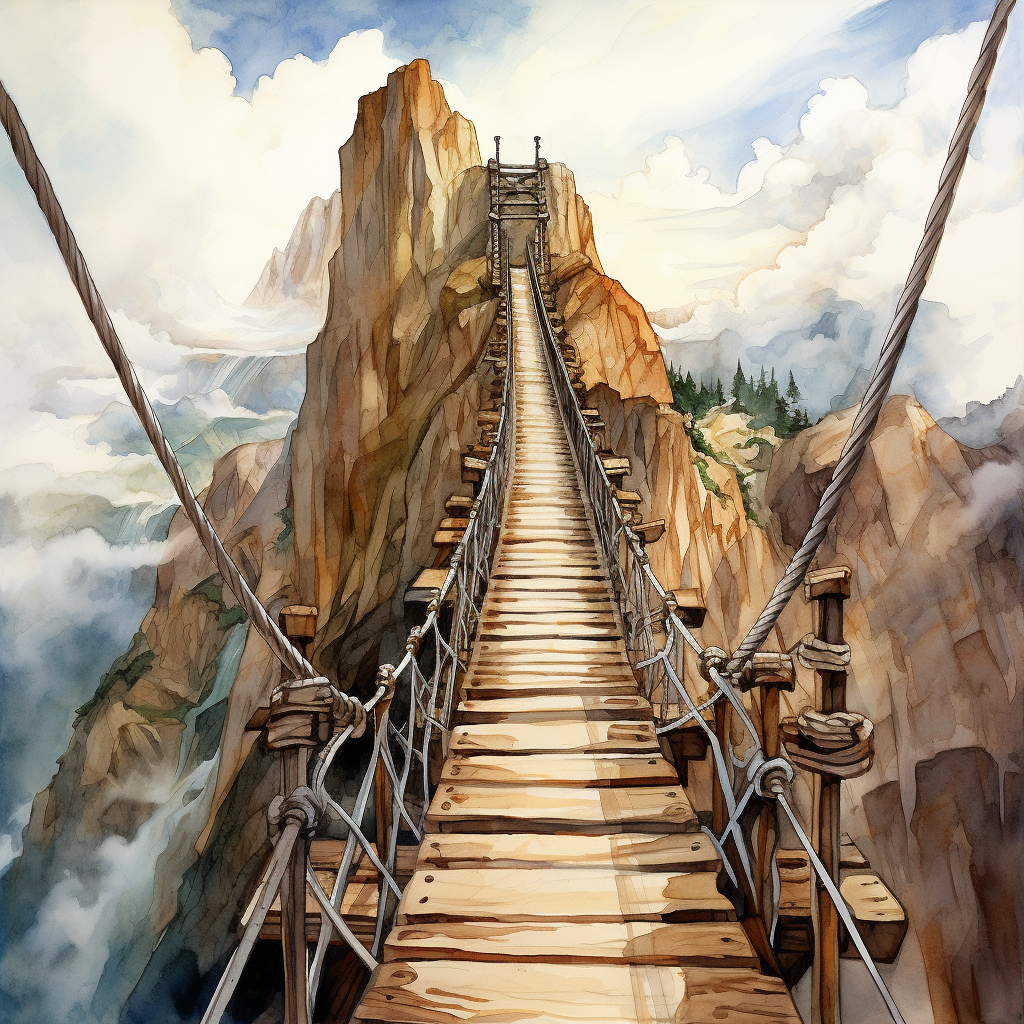
Illuminating answer (that matches what I am hearing from others) about why AIs don’t come with release notes: no one knows well what new models are capable of doing well or badly in advance.
It is why it is so important to test on your own use cases. Don’t just listen to others.
The problem (or at least one of the problems) is that the twin edicts to simultaneously optimize your team and life and to be flexible in light of an uncertain future are in opposition to each other. Optimization presumes a kind of certainty about the circumstances one is optimizing for, but that certainty is, more often than not, illusory.
Mandy Brown • Against Optimization
But if you don't have a use case then having this sort of broad capability is not actually very useful. The only thing you should be doing is improving your operations and culture , and that will give you the ability to use AI if it ever becomes relevant. Everyone is talking about Retrieval Augmented Generation, but most companies don't actually ha... See more
mataroa.blog • I Will Fucking Piledrive You if You Mention AI Again
Too Much Efficiency Makes Everything Worse: Overfitting and the Strong Version of Goodhart's Law
Jascha Sohl-Dicksteinsohl-dickstein.github.ioMLEs are happy to delegate experiment tracking and execution work to ML experiment execution frameworks, such as Weights & Biases 3 , but prefer to choose subsequent experiments themselves. To be able to make informed choices of subsequent experiments to run, MLEs must maintain awareness of what they have tried and what they haven’t (Lg2 calls ... See more